During my recent engagement with a leading management consultancy firm, I discovered an issue that is quite common among complex organizations: over-customization in financial data management, data tagging and financial report creation. This over-customization often manifests itself in financial reporting views that ultimately fail to correspond to GL data hierarchies.
The issue is exacerbated by the use of Professional Service Automation (PSA) systems (such as those used to create project codes) to handle management reporting when there is no direct tie back to the general ledger. The mingling of different systems and relying on them in ways in which they were not intended has downstream consequences.
Let’s explore the importance of doing honest assessments of the soundness of the general ledger structure, associated dimensions and project management systems in service of financial reporting and decision-making for organizations of all sizes.
How PSA Software Impacts Financial Data Management
Professional services automation (PSA) is software used to help professional services companies (or more broadly, service delivery companies) with project and resource management, typically for projects that involve billable hours and where utilization rate is an important metric.
PSA is valuable in the following areas:
- Project management
- Billing
- Invoice processing
- Time reporting
- Payroll
When optimally deployed, PSA can boost profitability and identify areas of revenue leakage and margin degradation by improving overall operational visibility. Another way to think about it is that PSA serves as the bridge, or link, between the customer relationship management and finance management functions of an organization.
What are Data Dimensions Used for in Financial Reporting?
To explain why over-customization can be such a problem, we should go back to the general ledger, a finance organization’s official record of every business transaction, including accounts payable and receivable, assets, liabilities and income statement accounts. Advancements in technology have precipitated the shift away from static ledger keeping to dynamic models that can automate accounting processes and customize data structures.
A key ledger feature is the use of dimensions that classify GL accounts in different ways. Dimensions are numbered, with:
- Dimension 1 being a legal entity or subsidiary,
- Dimension 2 being the geographic location and
- Dimension 3 being a department or operating unit such as a customer segment or industry vertical.
Additional dimensions can be added later for further filtering and sourcing. This structure gives an organization flexibility as the business grows to either add new GL accounts to capture new transaction types or expand the existing dimension list for new products and services.
Related Content
Inherent Limitations of Dimensions in Financial Data Management
Some organizations will treat Dimension 3 (i.e., “Department”) as the complete view, with business leaders budgeting solely on this dimension level instead of the GL account level. This creates problems downstream with inaccurate reporting of actuals versus budget and insufficient variance explanations. As actuals hit GL-level accounts, it can become challenging to tie them to the appropriate dimensions that exist in the PSA system but do not exist in the GL itself.
How can you identify the account responsible for a variance if you never budgeted or forecasted at that level and instead used the non-finance PSA system? It is either impossible or requires extensive and time-consuming investigative digging by individuals in both the finance and accounting departments.
I have seen cases where budget owners live entirely in the PSA world and do not even have access to select individual GL accounts during budgeting and forecasting exercises. These budget owners budget with PSA tools (which by their nature are not finance tools) by selecting revenue and expense types instead of actual GLA codes. The project codes they budget against then become the key translator between the PSA and financial reporting systems. Data elements are missing, however, as not all data populated in the financial system is present in the PSA system, and vice versa.
Collection of Data Elements: Is There a Clear Business Reason?
Certain data elements may be available in the system for tagging, but there should be a good business reason for their inclusion. One example would be the tagging of sales data to geographic regions. Most companies that utilize geographic tags have a strong regional component to the business, with regional budgets, goals and leaders who oversee specific territories.
Regional activity matters in those cases as geography has financial reporting value. But if the company is not organized in such a manner, and is instead centralized in nature, using a regional dimension may not be warranted and may only serve to complicate data organization. Companies should consider phasing out unused dimensions with the understanding that they can always be brought back later should they become valuable.
Financial Data Management: Time for a Routine Checkup
Fixing suboptimal data structures out of which all financial reporting flows requires an organization to interview the constituents who touch the process at any point (e.g., financial analysts, accountants, controller, budget owners) and leverage those learnings to map out the structure, systems and downstream flow of business information.
A finance organization that follows best practices for data management at one point in time cannot automatically expect to remain so indefinitely without checkups. An organization should conduct a complete audit of its finance and accounting data flow every few years to:
- Document beginning and ending points
- Identify pain points
- Identify lapses in best practices
- Make recommendations for improvement
- Implement those recommendations
As the business inevitably evolves and end-focus changes, the way sales data are captured, structured, allocated and reported will need to keep up. Naturally, they fall behind. When this happens, an organization’s mantra all too often becomes “this is just how we’ve always done things.” I’ve seen it time and again.
Learn More
The recommendations for improvement should be compared to best practices for the company’s particular industry to identify shortcomings. Some shortcomings, or gaps, in data management might be acceptable if justified for legitimate business reasons. Others are problematic or will soon become problematic if not quickly addressed, compounding over time until eventually few people in the organization understand the complete end-to-end data flow that touches all areas of finance, including budgeting, forecasting, and reporting. An outside finance consultant experienced in best practices for data management and financial reporting can prove invaluable in this effort.
For readers who suspect their accounting departments might have areas of improvement but aren’t sure how best-in-class departments operate, we also offer this helpful health-check guide:
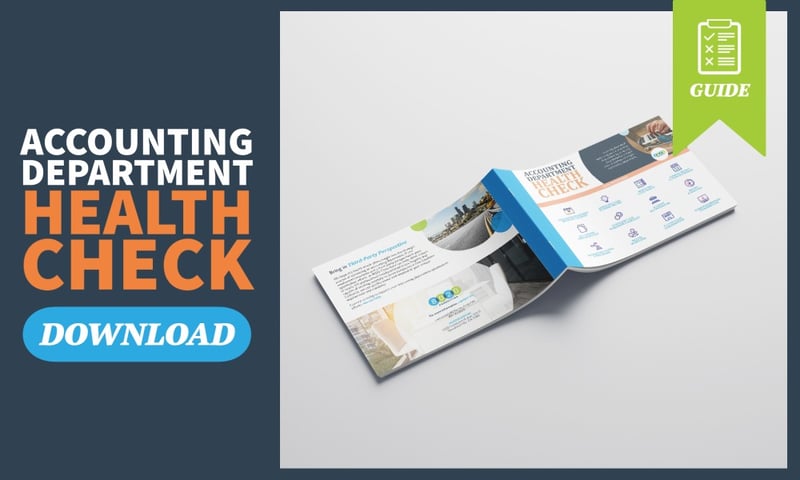